The results of the project; "AI based risk model for vegetation near power lines" can contribute to a reduction in the number of power outages due to vegetation, and ensure more cost-efficient vegetation management near power lines.
New Innovation Project for the Industrial Sector
In April 2020, the innovation Project for the Industrial Sector (IPN) named “AI based risk model for vegetation near power lines” was kicked-off. The project is funded by the Research Council of Norway (RCN), through the Energix program, funding innovation and research within sustainable development of energy systems.
The project contributes to UN Sustainable Development Goal no 9 through building resilient infrastructure, promoting sustainable industrialization and foster innovation.
The project results can contribute to a reduction in the number of power outages due to vegetation and ensure more cost-efficient vegetation management near power lines.
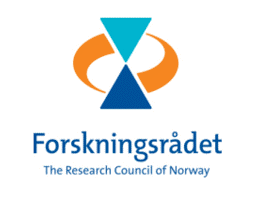

The project proposal feedback from RCN was:
“The project has a good consortium and ambitious goals that can be demanding to realize. The value creation potential is considered significant"
Project facts
- Project number: 309307
- Project period: 04-2020 to 06-2022
- Budget: 11 mill NOK
- Funding granted: 4.4 mill NOK
- Project responsible: eSmart Systems AS
- Partners: NIBIO (Norwegian Institute of Bioeconomy Research), Norgesnett AS, Valdres Energi Nett AS, Dalane Energi Nett AS, Ringerikskraft Nett AS, Gudbrandsdal Energi Nett AS
- Project description at RCN
Project goal
To develop an operational software solution for assessment of vegetation safety near power line spans, that utilities can use for prioritization and planning, based on local data on growth conditions and vegetation.
Challenge
Vegetation is the single most common power outage cause in Norway and many other countries worldwide. Outages are costly, both for the utilities, that get financial penalties, and for society. Electricity is needed to support most societal functions and modern societies are vulnerable to power outages and the preparedness level in the population is generally low.
As an example, in 2019, a five-day nationwide blackout in Venezuela, hitting 30 million people, caused the death of more than 23 people due to lack of electricity in hospitals. Pumps powering running water stopped and food spoiled in fridges.
An example of outage due to clearance issues is the 2003 Italy blackout caused by a tree flashover due to insufficient trimming of bushes and trees below high voltage overhead lines. The cascading effect disrupted power flow to Italy from the two main exporting countries France and Switzerland. Throughout Italy, 110 trains were cancelled, with 30,000 people stranded on trains in the railway network. All flights in Italy were also cancelled. Police described the scene as chaotic but there were no serious accidents. After three hours, energy was restored in northern regions. Electricity was restored gradually in most places, and in most cities, electricity was powered on again during the morning. Rolling blackouts continued to affect about 5% of the population on the next two days.
The same year, the Northeast blackout of 2003 in the US took place. The blackout's proximate cause was a software bug in the alarm system at the control room of FirstEnergy, an Akron, Ohio–based company, which rendered operators unaware of the need to redistribute load after overloaded transmission lines drooped into foliage. What should have been a manageable local blackout cascaded into the collapse of the entire Northeast region. In some areas, power was out for 4 days, affecting in total 45 million people in 8 US States.
One of the conclusions from the investigation was:
"FirstEnergy failed to manage adequately tree growth in its transmission rights-of-way."
High costs due to clearance issues
The utilities in the project all report high costs related to both financial penalties due to vegetation related outages and vegetation management. Overall, more than 50 % of total financial penalties are due to vegetation related outages. The costs related to expensive vegetation management regimes are almost twice the financial penalties related to vegetation, and increasing.
Pacific Gas & Electric (PG&E), one of the largest utilities in California, cuts or prunes 1,4 million trees every year. In the period 2013-2018 they spent 1,6 billion USD on vegetation management. To prevent the disastrous wildfires in California, a high priority is enhanced vegetation management.
Climate change
Climate changes cause more extreme weather and a higher vegetation growth rate due to increased CO2 levels in the atmosphere. Wind directions can change, affecting trees that are hardened for one wind direction, but not for another. Stronger winds will occur more frequent, snow will be more humid and heavy and lack of frost in the ground makes vegetation less stable. An increased risk of draught might weaken trees. Thus, the risk of vegetation related outages is expected to increase with climate change, enhancing the need for utilities and society to handle vegetation risks more efficiently.
Utilities’ current vegetation management strategy
Utilities spend considerable time and money on vegetation management, often based on simple and costly strategies such as vegetation clearance of power corridors at regular intervals.
This strategy might result in removing stable trees that protect the power lines from less stable vegetation, thereby exposing the unstable trees and possibly increasing the vegetation growth rate near power lines due to an increase in light. In addition, the strategy might be blind to local factors affecting the vegetation growth and vegetation risk.
Lines are inspected by foot patrol, by helicopter/UAV 2D images or by Lidar inspections. This provides some insight into the complex problem of vegetation risk, mostly related to the distance between vegetation and power lines, particularly where clearcutting is not an option (difficult terrain, landowner, or regulatory disputes etc).
Lidar, the current business as usual approach for identifying vegetation risks in the power grid, can be used to identify vegetation close to the network and provide a static snapshot of the state of vegetation threats. This provides some value, but does not provide the valuable and cost-effective prioritization that can easily be done by also taking into account the stability of vegetation defined by knowledge of local vegetation properties and the local soil/ground conditions.
In order to have fully tree safe power lines in Norway, where trees can grow to 30m height, the width of the clear-cut corridors must be up to 40-60m, which is practically and economically impossible in most cases.
Lack of competence on forestry and vegetation risk assessment
Many utilities lack the needed competence on forestry to make proper assessment of which vegetation poses a risk to the power lines and why it might constitute a risk, leaving the utilities to perform simplified assessments at high costs. One simply cannot expect the traditional manual processes to fully prevent vegetation threats.
Proposed solution
Research has shown that properties of the trees, terrain, soil type and climatic conditions are predictive for the probability of treefall, one of the major and most unpredictable vegetation threats. By targeting trees or stands at risk, beyond simple distance-based Lidar analysis or clearcutting of corridors, the utilities can perform more efficient vegetation management. Combining the probability of a treefall with the consequence of a treefall at span level in the grid, the utilities can plan and prioritize vegetation management more efficiently than with current practices.
The cost-saving potential is estimated to be 20-40 % for the vegetation management costs. In addition, reduced penalties can be expected. The overall cost saving potential is large.
Figure 1: Example of vegetation risk management software
Figure 2: Example of vegetation risk management software
How? The probability and consequence of treefall must be taken into consideration
There are many factors that impact the overall risk of treefall. Properties of the vegetation, such as tree type, tree condition and tree shape impact the probability of a treefall. In addition, the local growth conditions such as topography, soil conditions and typical climate will also impact the probability of a treefall. A healthy tree located in good soil, with deep roots and usually exposed to heavy winds from north, will most likely not be impacted by a storm from the north due to hardening. As an opposite example, an old and spruce, located in wet terrain and with shallow roots, will more likely be impacted by a heavy storm and should be removed if not at safe distance from the power line.
In addition to the probability, the consequence of a treefall must be estimated to properly evaluate the overall risk. For instance, if a line has many switches or there are only holiday homes connected, the consequence of a treefall will be lower than if the power cannot be routed elsewhere and critical customers or contracts are impacted.
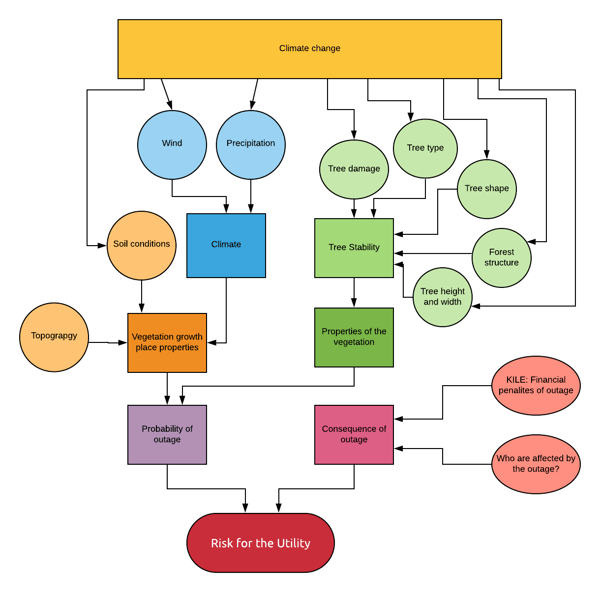
Project research hypothesis
Using a combination of existing data sources as DTM (digital terrain models), satellite-based forest maps and similar, combined with direct and «fresh» measurements (RGB images or other sensors) using UAVs and processing of these using AI, can make vegetation risk models near power lines much more precise.
The project aims to fill a knowledge gap about:
-
How to use AI and image recognition to determine local tree specific variables from RGB images or other sensors
-
How to best combine heterogenous data sources to determine probability of treefall
-
How to combine the probability of treefall with the consequence of a treefall to an operational risk model
-
How to develop architecture and data models that can handle different and heterogenous data sources for the purpose in a software
Figure 4: Example of AI capability to identify classes of trees
Project status
During summer 2020, the project will do its first, of two, round of data collection, followed by the development of AI models for detection of tree-specific parameters in late 2020 and beginning of 2021. Development of risk models and data models will also start in 2020.
Contact information
-
- eSmart Systems - Project manager: Kathrin Sunde
- eSmart Systems - Chief Analytics Officer: Davide Roverso
- eSmart Systems - Chief Solutions Architect: Christian Thun Eriksen
- NIBIO-Senior researcher: Svein Solberg
- Norgesnett - Manager Grid Development: Vidar Kristoffersen
- Dalane Energi Nett - Manager Grid Operations and Maintenace: Bjørn Sviggum
- Ringerikskraft Nett - Electrical Power engineer: Irina Pirozhinskaia
- Gudbrandsdal Energi Nett - Forestry agent: August Johan Evensen
- Valdres Energi Nett - Manager Grid Operations and Maintenace: Svein Hegge